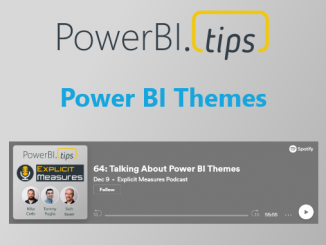
Power BI Themes
Themes are the bedrock of consistency. As report authors it is important to create a consistent experience in a single, series or multitude of reports. With a little forethought you can easily build reports that […]
Themes are the bedrock of consistency. As report authors it is important to create a consistent experience in a single, series or multitude of reports. With a little forethought you can easily build reports that […]
First of all, go read this amazing blog put out by Alberto Ferrari over at SQLBI that he posted awhile ago. It is the context for the conversation we had and is well worth the […]
This month we partnered with the relaunch of the Des Moines user group to host Matthew Roche. This month’s topic is all about data culture. If you don’t know Matthew you should get to know […]
This topic was gleaned from the absolute wealth of knowledge put down in the MSFT Power BI Adoption Roadmap. We highly recommend you check the full roadmap. In this episode, we focused in on Data […]
To say this another way. Not all columns have datatypes in DAX, specifically speaking to using dynamic ranking with an “Other” category. Let me explain. The Tabular model is comprised of tables with typed columns. […]
Trolling… we should clear up the definition of this right off the bat. We aren’t talking about the type of trolling where we spend a bunch of time finding things on the Power BI Ideas […]
Copyright © 2024 | MH Magazine WordPress Theme by MH Themes